Fasten your seatbelts. It’s going to be a bumpy night.
As an Oscars diehard, Ben Zauzmer surely recognizes that line from All About Eve, tied as the most heavily nominated film in Academy Awards history. As a data scientist who built a predictive model to forecast Oscar winners each year ahead of the awards show, he surely sympathizes with the sentiment too.
Recent after-effects of the pandemic have thrown a wrench into Zauzmer’s carefully calibrated formula. Film festivals that normally generate important buzz for films could be either canceled or staged virtually. Releases have been delayed as theaters shuttered. And the Academy made the unprecedented announcement in late April that streaming-only films will be eligible this year. There’s even been a major, non-pandemic-related recent change: The Academy combined Best Sound Editing and Best Sound Mixing into a single category, Best Sound. (As if you knew the difference anyway.)
“This is a situation we’ve really never had in the history of the Academy Awards,” said Zauzmer, who by day manages baseball analytics for the Los Angeles Dodgers.
The host of volatile variables complicates what was already difficult data analysis. Yes, the Oscars date back decades, but there’s still a paucity of data, relatively speaking, and that makes prediction modeling very difficult.
“I’ve often joked that we sometimes have more data on a single [baseball] pitch than we have on the entire history of the Oscars. It’s a joke, but it’s also not a joke.”
“The Oscars are a game of small sample size,” said Zauzmer, who last year released the book Oscarmetrics: The Math Behind the Biggest Night in Hollywood and writes about his predictions for the Hollywood Reporter. “I’ve often joked that we sometimes have more data on a single pitch than we have on the entire history of the Oscars. It’s a joke, but it’s also not a joke.”
It’s safe to say that Zauzmer’s model will face a challenge unlike any other since he started the project in earnest, in 2012, as a Harvard University freshman. We spoke with Zauzmer about how (if?) he’ll be able to adjust his model for such instability, what effect streaming might have on voting, whether he’s able to account for the Academy’s recent attempts to expand membership in his formula, and more.
Do you think any of these wild variables caused by the pandemic will significantly affect your model? Do you plan on adjusting it?
At this point there might be more unknowns than knowns about how Oscar season will play out.
That said, when it comes specifically to my model, the single most important factor is the other award shows. Other factors go in, but those previous awards shows — whether they’re similar organizations like the Golden Globes or British Academy Film Awards (BAFTAs) or the various guild awards — tend to be among the most predictive indicators. So how accurately I’ll be able to predict the Oscars will largely come down to how much the Academy and these other predictors stay aligned.
That doesn’t just mean aligned in choosing winners, though that’s part of it. It could be that if all viewing is online, maybe that helps certain films, or makes some voters more likely to vote in ways that are difficult to predict, since we’ve never had it like this before.
It’s also a question of how much they align on the calendar. If, hypothetically, things are so out of whack that some guild awards take place after the Oscars, or not at all, that would throw a serious wrench into the way I predict the Oscars.
We don’t know, at this point, of any of the major winter awards that have moved from their traditional dates, but I’m sure they’re all considering various contingency plans. It could be a possibility, depending on how bad the pandemic still is at that time.
You mentioned streaming. There’s no way to know how many nominated movies voters actually watch. But it would seem that if some movies debut on streaming, and people are less busy, voters might see more nominees. Could that affect the model?
Ironically, people not being able to go out — if that is still the situation when Oscar voting occurs in January — could actually lead to a more fair Oscars.
I’ve found in my research a strong trend between being nominated for Best Picture and winning other categories, even categories like Best Makeup and Hairstyling, which seemingly would have little to do with Best Picture. The general explanation is that voters are simply more likely to have seen Best Picture nominees. And if you’re more likely to have seen a movie, you’re more likely to vote for it. So you could have a situation where more voters are able to see more movies. If that happens, it’s more fair.
“I’ve found in my research a strong trend between being nominated for Best Picture and winning other categories [that] seemingly would have little to do with Best Picture.”
That is pretty difficult to account for in my model because right now there’s a boost in every category for being in a Best Picture-nominated film. And the question is, should that be lowered in a year where voters are able to watch more movies? And if so, how much?
The second question almost answers the first. Because I don’t know how much to lower it, because we’ve never done the Oscars in a pandemic, I really can’t lower it. The vow I make is to not pick any coefficients or constants or weights myself. I let the math decide it all. And the way the math decides these things is based on past years’ data. If past years are not representative because of a global pandemic, then that would definitely skew the model.
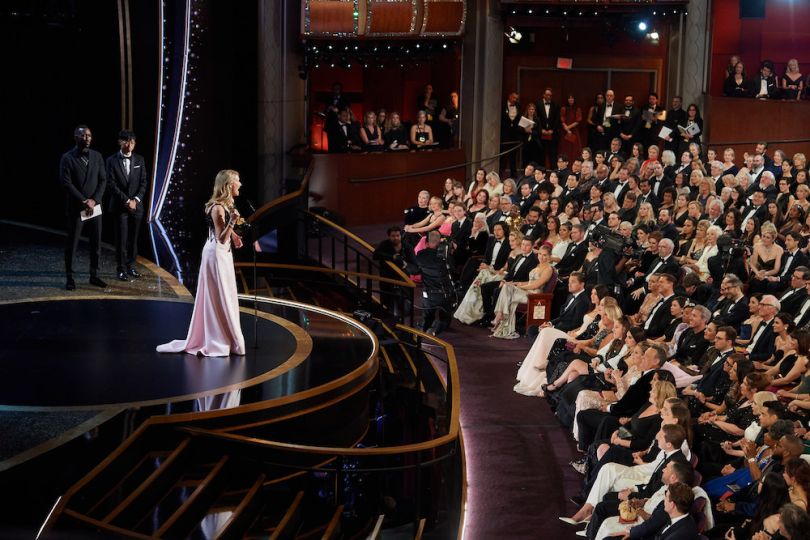
How predictive is a film’s popularity? I ask because Netflix is opaque about viewership totals, which could be significant if it releases its major contenders only, or primarily, to streaming.
I kind of lucked out with box office. When I started doing this, in 2012, I didn’t face a situation where some movies weren’t releasing box office numbers, because Netflix wasn’t yet competing at the Oscars. Every movie had box office numbers. And while it is in the model, it turns out it’s just not that strong a factor.
Now we’re living in a world where Netflix competes at the Oscars and some of the movies simply don’t release box office totals. So if the model heavily relied on those, I would be up a creek. Some major contenders are not offering the same level of data that more traditionally released films are.
The two sound categories have been combined into one. How will your model account for that?
It’s funny — the merger was announced the same day the Academy announced new eligibility requirements, at least for this year, that allow streaming-only movies to qualify. In Hollywood, [the streaming news] was the big deal. But to me, as Oscars predictor, the bigger deal was the sound merger.
I’ve got my model for Best Sound Editing and I’ve got my model for Best Sound Mixing, but I had no model for Best Sound. While some predictors lean more toward one or the other, there’s no real way to know how much to weight those two things relative to each other. Will these new voters be more inclined to lean toward sound mixing or sound editing? Does every voter have a firm grasp on the difference? If not, is that part of the reason they’ve combined?
We do know a little bit by way of the BAFTAs. They tend to be predictive of the Oscars in some respects, and they’ve had one combined sound category for a long time. The BAFTAs tend to be a little bit more aligned with sound mixing than sound editing, so that’s some evidence that perhaps the Academy will do the same. But that’s really just educated speculation, because we haven’t seen a combined best sound category at the Oscars in many years.
How much are you able to account for small sample size? Maybe there’s a relatively new awards show, or more recent data appears more predictive than older data from, say, the 1930s?
I do precisely that.
When it comes to a small sample in the dependent variable, there’s little a predictor can do about that. So if I had been predicting the Oscars the year Best Animated Feature debuted, I very likely would have simply skipped the category, since there was no history at all. That makes it nearly impossible to properly weight the different potential factors without using some guesswork as to which things are more predictive. My whole shtick is that I don’t use guesswork — it’s all based on a dispassionate statistical view of the historical record.
When it comes to small sample sizes in the independent variables, I’m able to do some things. There are times when, after just a few years, a new award show has a decent track record of predicting a given category. In a game of such small sample sizes, it’s really painful to throw out anything that even might have some chance of helping predict the category. So yes, I’ll try to weight more recent data more heavily. I’ll give more weight to predictors that have been around longer and have shown a longer sustained track record of success. And do this in a way that’s consistent across categories, so that I’m not just picking weights.
But inevitably in any model, even if I’m not picking weights, it’s fair to say that I’m making model selections. I’m making educated assumptions about how the general structure of the model should go. That’s just unavoidable. You can’t use a blind machine learning algorithm and just plug everything in and hope for the best when you’re dealing with such small samples.
“It’s fair to say that I’m making model selections.... You can’t use a blind machine learning algorithm and just plug everything in and hope for the best when you’re dealing with such small samples.”
You do have to make some general assumptions about how these variables interact or don’t interact and how more recent data should matter more. And then you let the math tell you how much you should weight higher-sample data over lower-sample data, and more recent data over older data. Then within the structure of the model you’ve built, you hope the math is at least able to come up with the best one within that universe.
At what point would you know you had enough historical data to make a good-faith prediction for a newer category?
I’ve never predicted a category at the Oscars using less than 10 years’ worth of data. But there’s nothing magical about 10. That’s as much a display choice as it is mathematical. Here’s what I mean by that: If you made a prediction in the very first year of a category, and there were five nominees, you would simply get a result of 20 percent for all five nominees, because you’d have no historical record.
Then the following year, when you have one year’s worth of historical data, and assuming you haven’t built some sort of overfitted model, but one that actually properly handles small sample sizes, maybe the leader would be at 23 percent and the last-place movie would be at 17 percent. They’d all still be bunched together.
As years go by and the data learns more about what’s predictive and what’s not, the leader would get higher, and the last-place movie would start to fall into the single digits.
So I wait until the numbers have a chance to spread out. But there is no magic answer as to when the math is confident.
Because we’ve had this period of category stability at the Oscars, this hasn’t been an issue for me. The newest category [Best Animated Feature] was already a decade old when I got started in depth. If they were to introduce Best Popular Film, for instance, that’s where the decision starts to become really interesting. A lot of people would ask me on Twitter for a prediction in the category, even in the early years of it.
I’d have to decide at some point, “OK, there’s enough data; it’s time to go on the record with this one.”
You have a number of things based not on statistics but on just domain knowledge — knowledge of how the Oscars and movies work. You do have a number of things that would give you a strong prior. You just don’t quite know how predictive it is relative to all the other things you think might be predictive.
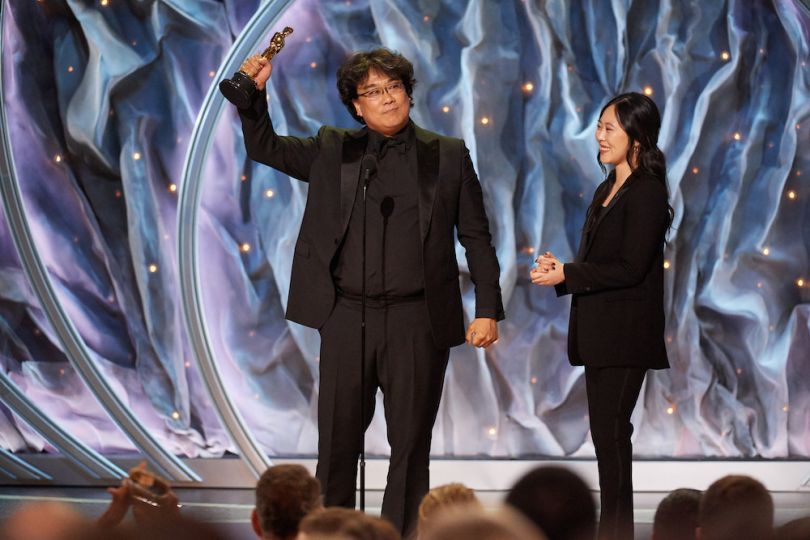
Do you have to adjust the model for new trends — I’m thinking of the recent split between Best Director and Best Picture — or is it built to handle new variations?
Over time, the model sort of does that itself. We’ve seen it with the Picture/Director split, and it’s a fascinating point. We’ve seen, for a number of years in a row, there has often been a film that will dominate throughout award season and win most of the major awards. Sometimes it’s a movie like The Shape of Water that goes on to win Best Picture. And sometimes it’s a movie like La La Land that goes on to lose, very memorably. But even though these movies tend to have similar Oscar resumes to each other, the percentage on the leader in my model has gone down, down, down, over the last few years. So even if you dominate everything — you win the Director’s Guild, the BAFTAs, the Golden Globes, the Producers Guild and so on — the odds of winning Best Picture have fallen.
The reason is exactly what you said, the math by itself is adjusting slowly to this new reality that just because you’re in line to win both Director and Picture, your odds of completing that sweep have gone down — because the last decade has seen fewer and fewer movies successfully make that sweep.
Over time, if that were to continue happening for, say, several decades, all Best Picture nominees would have identical chances of winning. Now, that’s unlikely to happen. I expect over the next decade that many of these traditional indicators will get back on track and continue to be good Best Picture predictors, and Best Director and Best Picture realign like they once were. But that’s speculation. It’s possible that we’ve entered a new normal where Best Director and Best Picture are not nearly as related as they were for the first eight decades of the Oscars.
Will the potential changes in the festival landscape alter your predictions?
I wrote a chapter in Oscarmetrics about film festivals and their relation, particularly to the Best International Feature Film. The main reason why film festivals tend to be weaker predictors is that they’re one of the few venues that are really big, media-wise, on the Oscar calendar that simply don’t have all the competitors going up against each other.
Something wins, but the other four movies in its category weren’t even there. Film festivals tend to be valuable not so much because they’re predicting the ultimate winner, but because they’re building buzz for who the eventual nominees are. So I do believe that if you have radically different film festivals, or no film festivals at all, it could change who the nominees end up being. That’s much more likely than the film festivals actually changing who the predicted winner is going to be after we already know the list of nominees.
You also predict who the nominees will be, too, correct?
I do. With that, I typically stick to just the eight biggest categories — picture, director, screenwriting and acting. And for those, film festivals have even weaker correlation. Best International Feature tends to be the most prominent of these, and Best Picture at least for generating early buzz. But I don’t expect that if, say, the Cannes Film Festival is entirely online or there is no festival at some of the other major sites, that that would have much meaningful impact on who was eventually nominated for Best Picture if everything else in Oscar season were continued as normal.
Now, if this continues into the fall or winter, and there’s a chain reaction — a film that would have gotten buzz in, say, Toronto doesn’t get that buzz and doesn’t continue on throughout Oscar season — we could be living in some sort of parallel universe where a movie that none of us went on to see actually would have been the Best Picture winner in a normal year. But it’s hard to know the unknowable.
The Academy has made recent attempts to expand its membership, and it’s now believed to be more diverse than before. Can you incorporate that change into the model?
I would love to be able to. The trouble is in the data. I co-wrote in the Hollywood Reporter a feature breaking down the expanded membership. And then separately I’ve written articles about predicting the Oscars. I’d love to have the ability to write an article about both, to get at exactly this question — how this new membership affects or doesn’t affect the results of the Oscars.
But to be able to answer that question, you need not just the final votes, but the individual votes — much like how many baseball writers publicly release their Hall of Fame ballots. I wish we had that, but we don’t. We have one piece of data from the Academy, which is who won the category — and that’s it. If we knew more about who was voting for whom, then maybe we’d be able to get a better sense of how or if the new membership affects final results.
Perhaps I’m biased because more data would lead to more interesting findings and make my job easier, all at the same time. But it would be a really fascinating data set.
In your book, you reference receiving an insider tip that voters were feeling The Artist fatigue and were starting to favor Hugo for Best Picture in 2011. It didn’t play out that way, and you didn’t let that information alter your model. But is there any way to quantify that kind of anecdotal information if it is reliable?
I have, and I think it would be enormously useful for the model. When you think about election modeling, it’s a combination of macro factors — how the economy is doing, overall approval rates — and more micro factors — talking to individual voters in different counties and swing states, gathering polls. Then a good election forecaster is able to combine the overall data and the individual polls and figure out the probability that each candidate wins.
It’d be amazing to do that for the Oscars. It would increase the accuracy of the model to combine things like Golden Globes and BAFTAs with individual polls of some subset of the Academy, then figure out the most mathematically savvy way of blending them.
The problem unfortunately is a resource one. Polls are difficult to organize and run. It’d be very difficult to orchestrate a poll of the Academy and get a meaningful number of responses. But perhaps one day, because I do believe, like you’re saying, it would add to the accuracy of the model.
Throwing out a whole bunch of statistical words isn’t even necessarily the best way to talk to fellow statisticians.
For the Oscars and baseball, a big part of your job is translating statistics for laypeople. Do you have any tips for fellow data practitioners?
It is such a big part of my job in baseball as well as what I do with the Oscars. Whether I’m talking to a coach with the Dodgers or writing an article, I try to talk more like a fan and put everything in terms of that domain. Throwing out a whole bunch of statistical words isn’t even necessarily the best way to talk to fellow statisticians.
My job is to communicate what I know, which is Oscars data and statistics, in a way that’s fun and interesting and based on stories instead of raw numbers. It’s not always easy. There are some concepts that are more easily translated using statistical terms. The goal is trying to put them in the vernacular of the domain without losing any accuracy that could come from straying away from quantitative terminology.